Research Center for Computing and Multimedia Studies, Hosei University, Japan
This paper proposes a speedup convolutional neural network (CNN)-based deblurring framework (SpeedDeblur) for reconstructed blurry videos. First, we extract the coding information and the reconstructed video from the compressed data. Second, a CNN-based algorithm is used for deblurring the first reconstructed frame. Pixels of the deblurred frames are transferred to the subsequent frames guided by HEVC decoded data.The transferring process is simple and faster than applying a deblurring algorithm on all frames. However, passing pixels throughout a long video propagates accumulated errors and reduces the deblurring performance. To bridge this gap, we design an adaptive reset strategy for deciding which frame needs CNN-based deblur during the transfering process. Besides, a data generation strategy simulating blurry real-world factors such as camera shake and fast movement is proposed. Compared to frame-by-frame deblurring approaches, our framework can retain the same comparable results and boost the deblurring processing by up to 4.0 times and 99.4 times on GPU and CPU, respectively.
Techniques :
Video Compression
Video Deblurring,
Frame Interpolation,
Convolutional Neural network
Programming Languages and frameworks :
Python,
MATLAB,
PRESENTATION VIDEO
PRESENTATION IMAGES
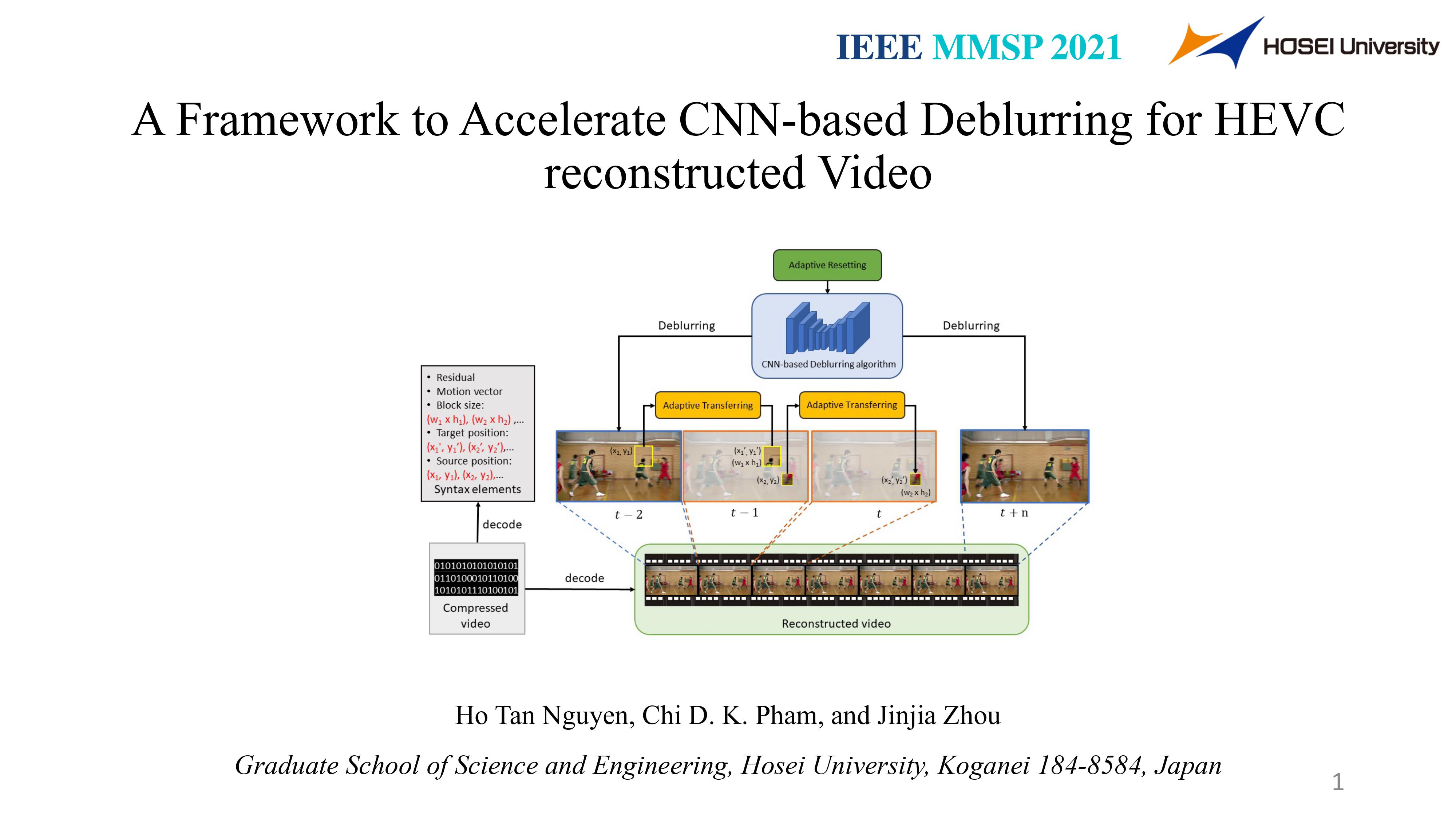
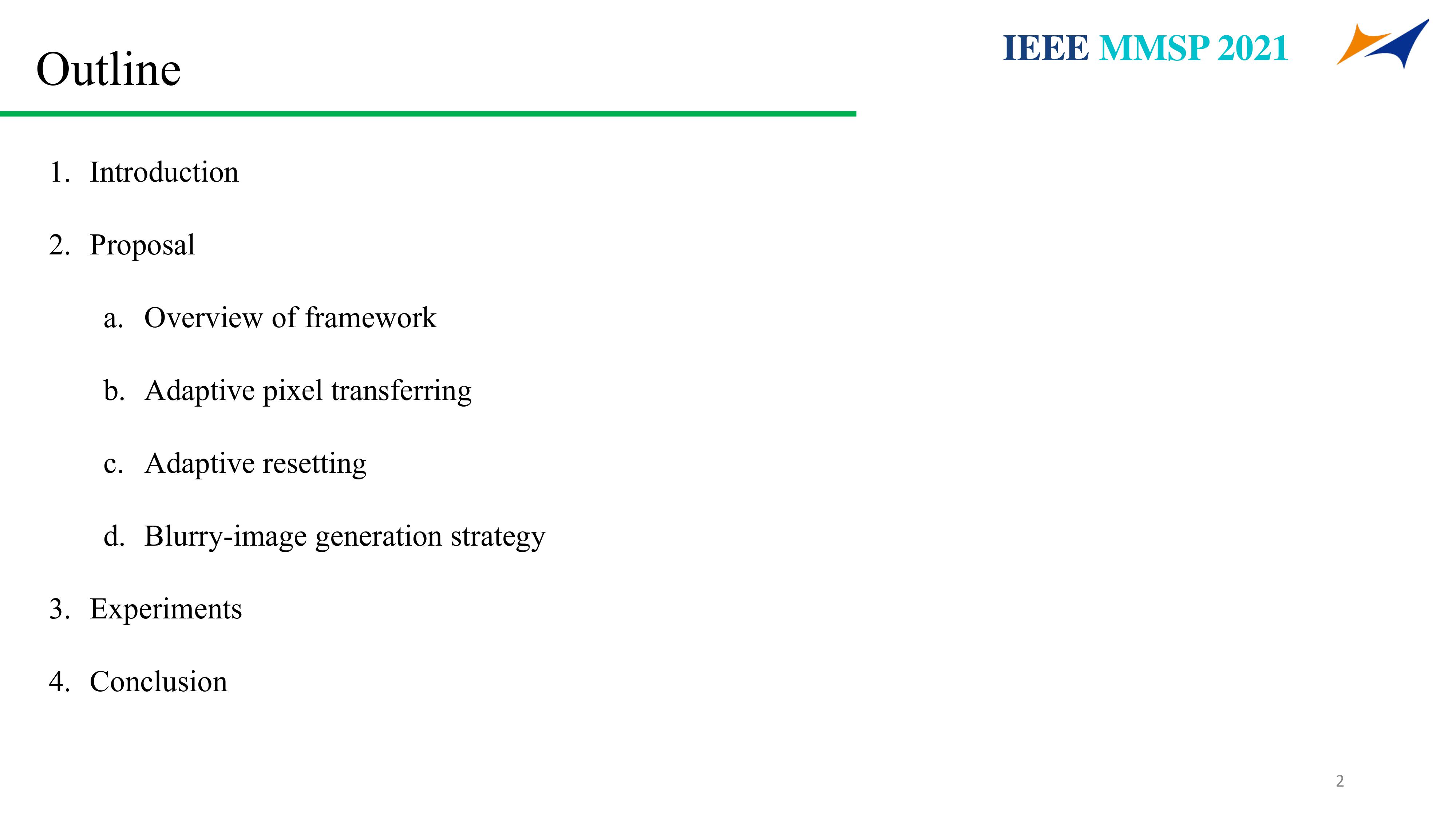
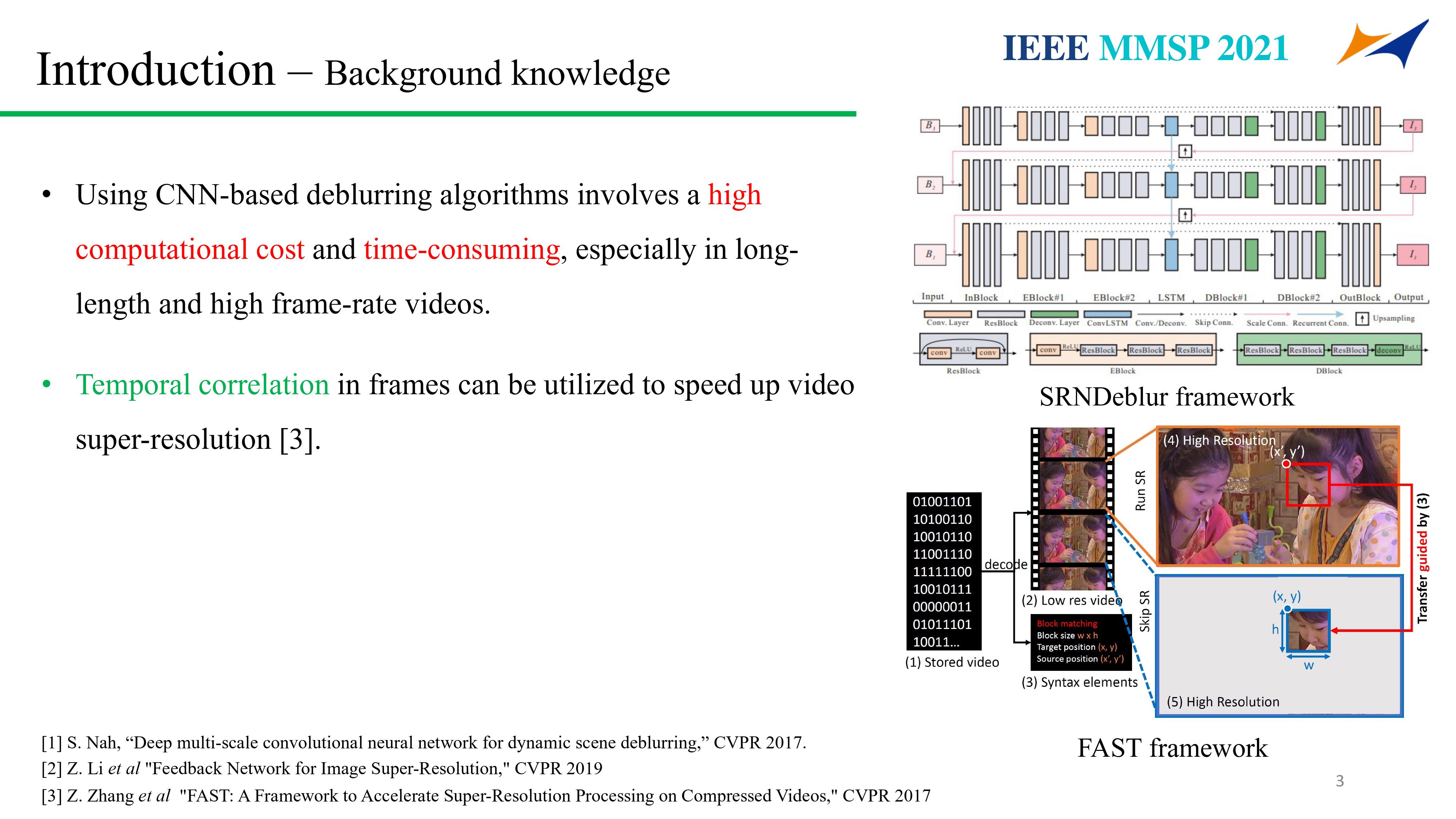
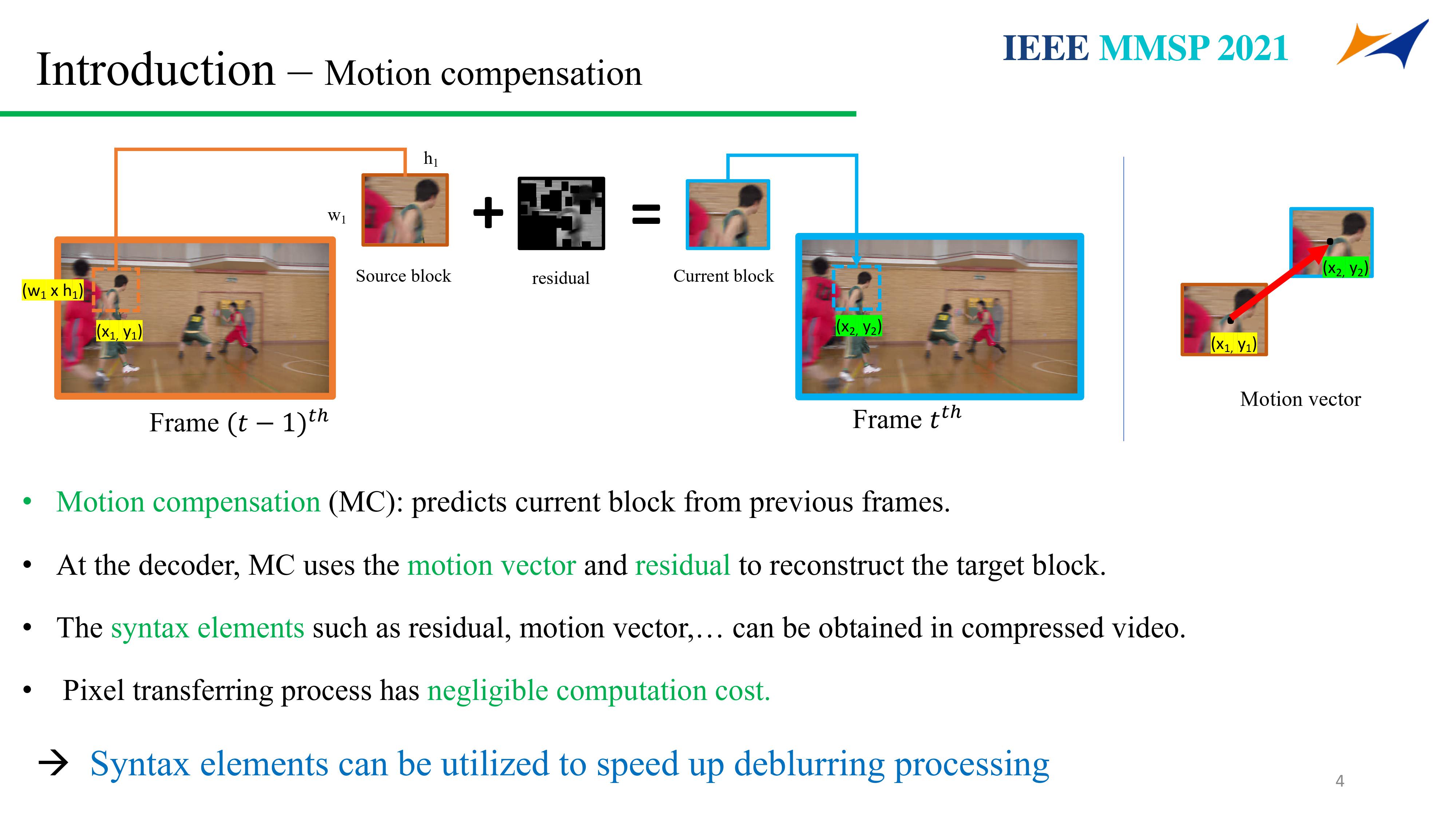
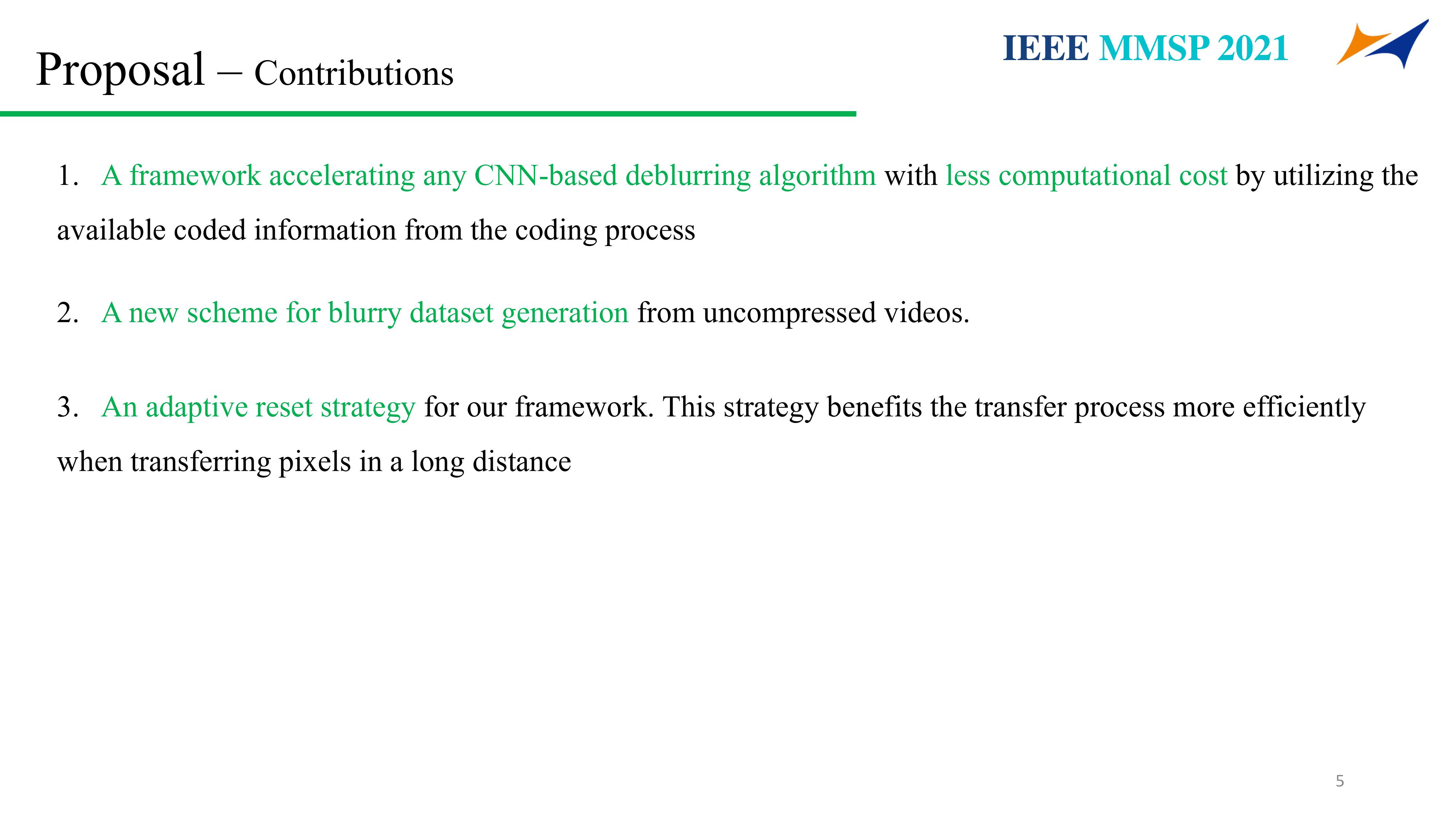
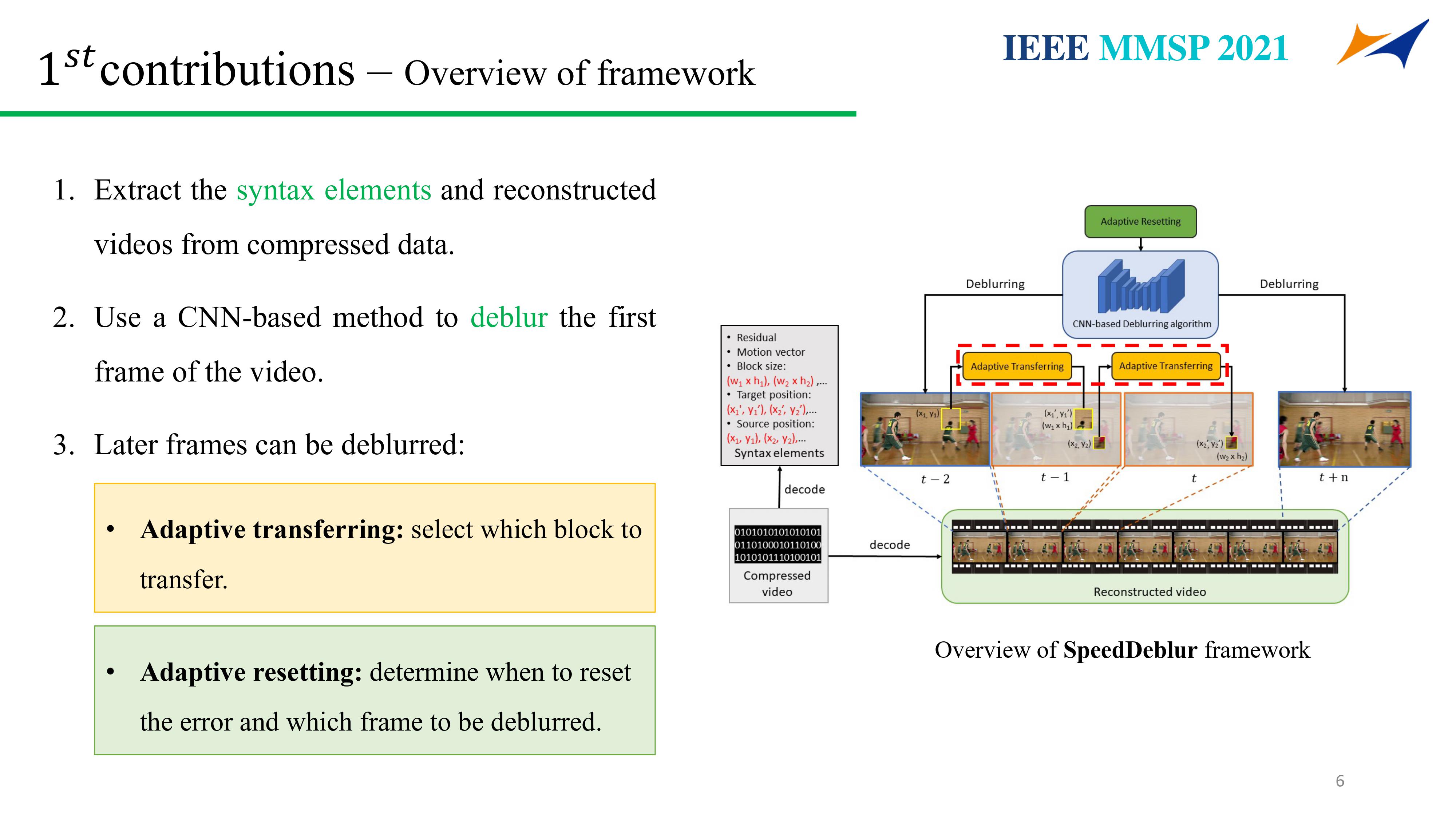
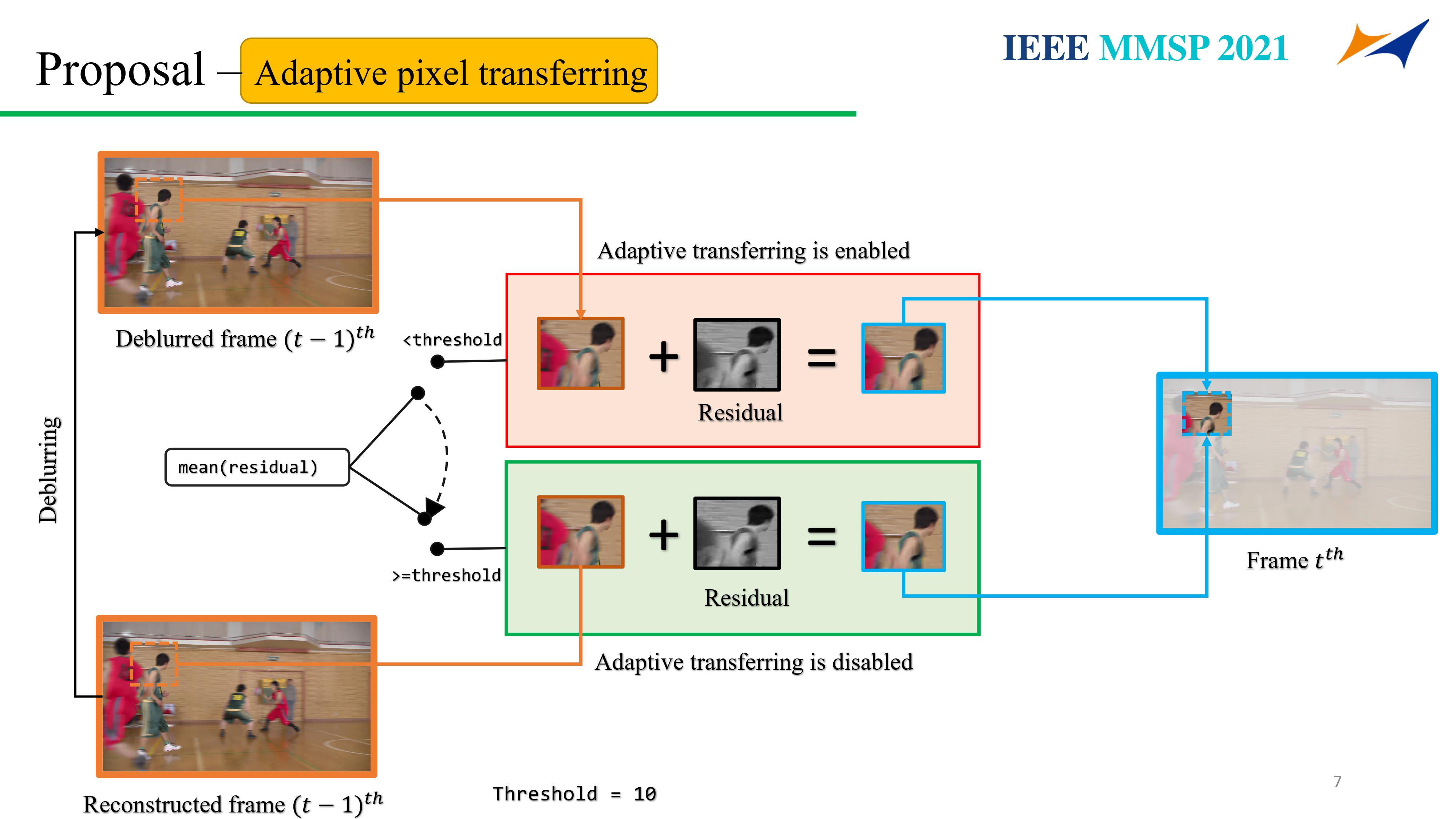
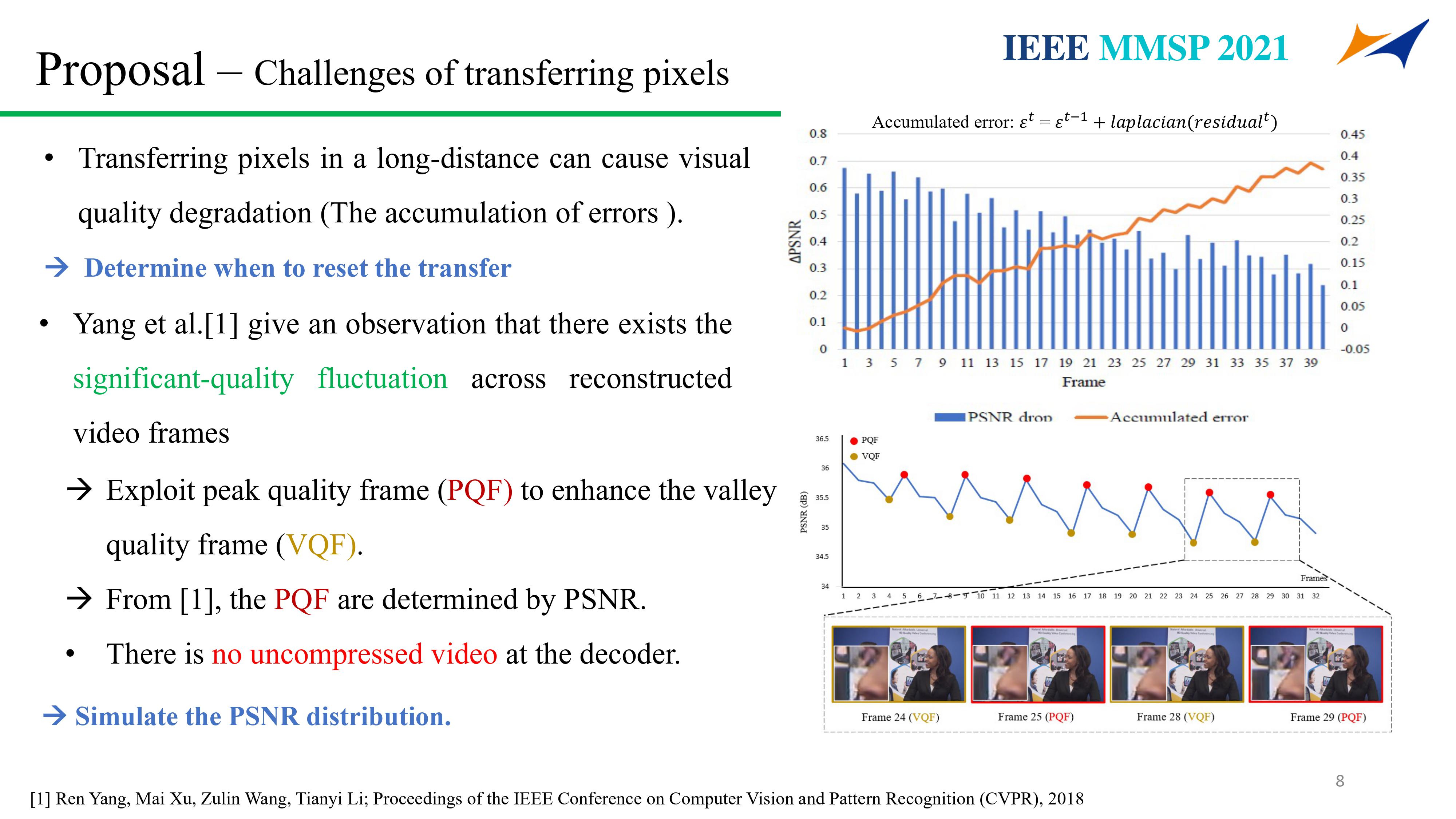
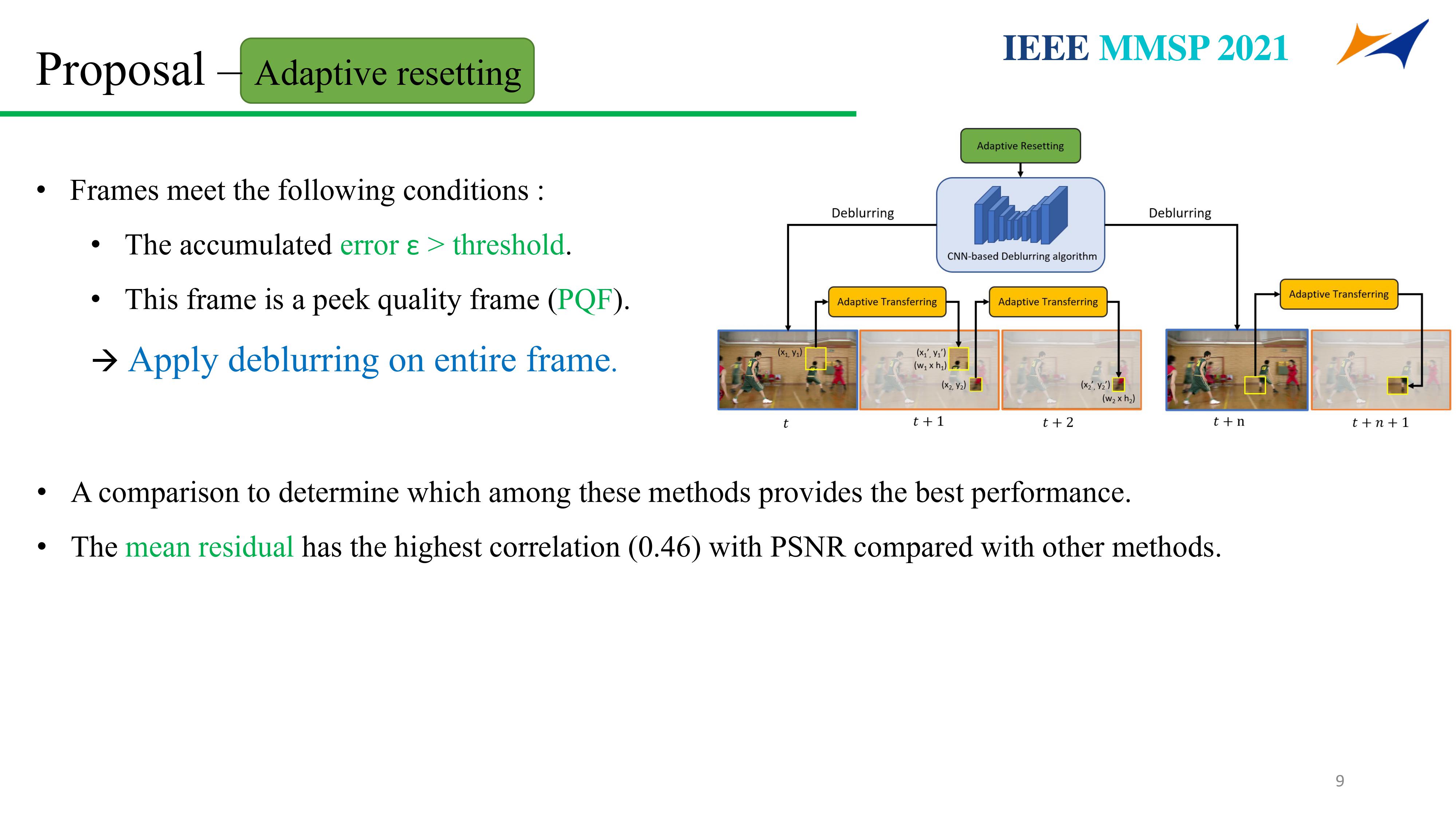
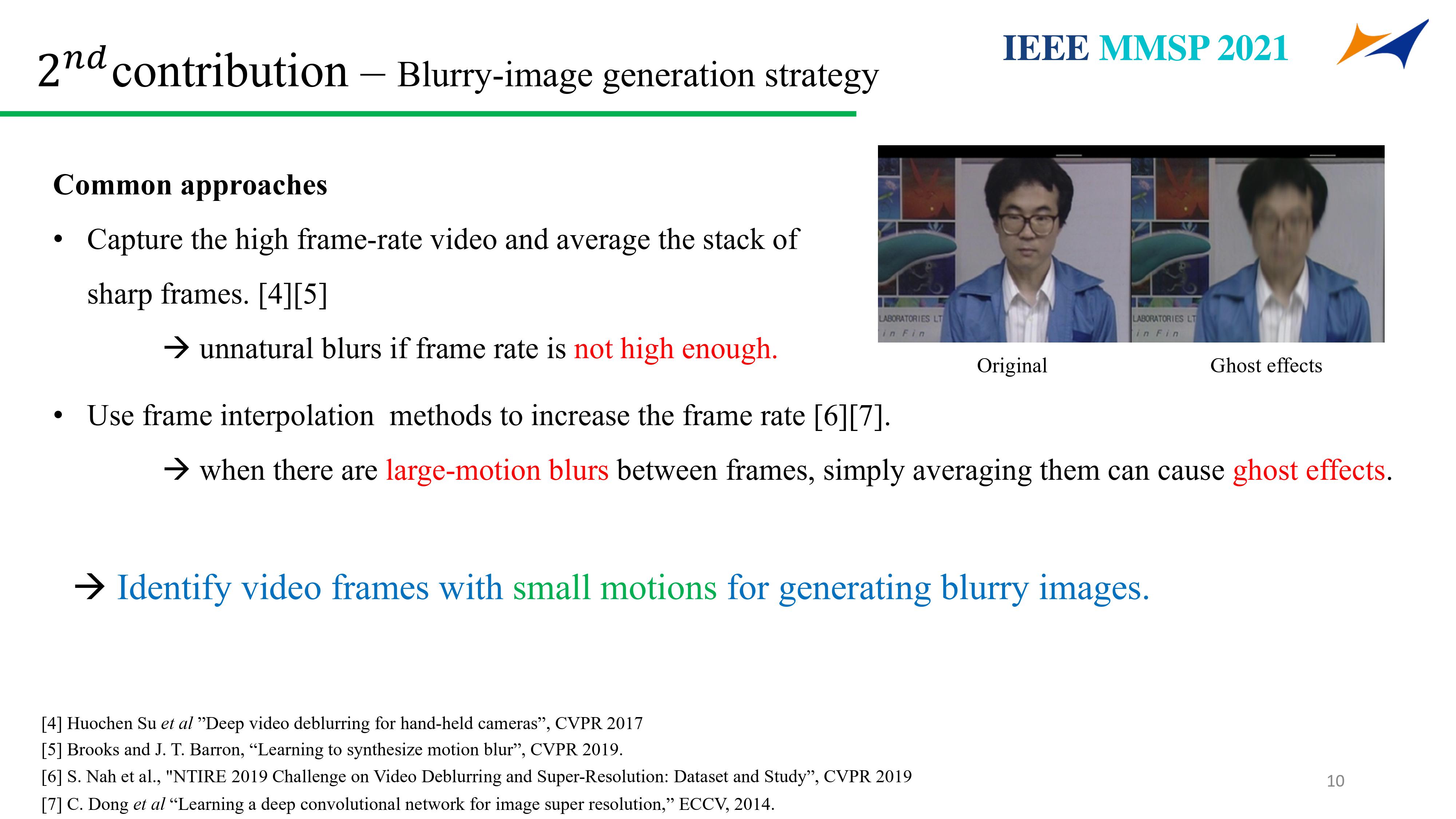
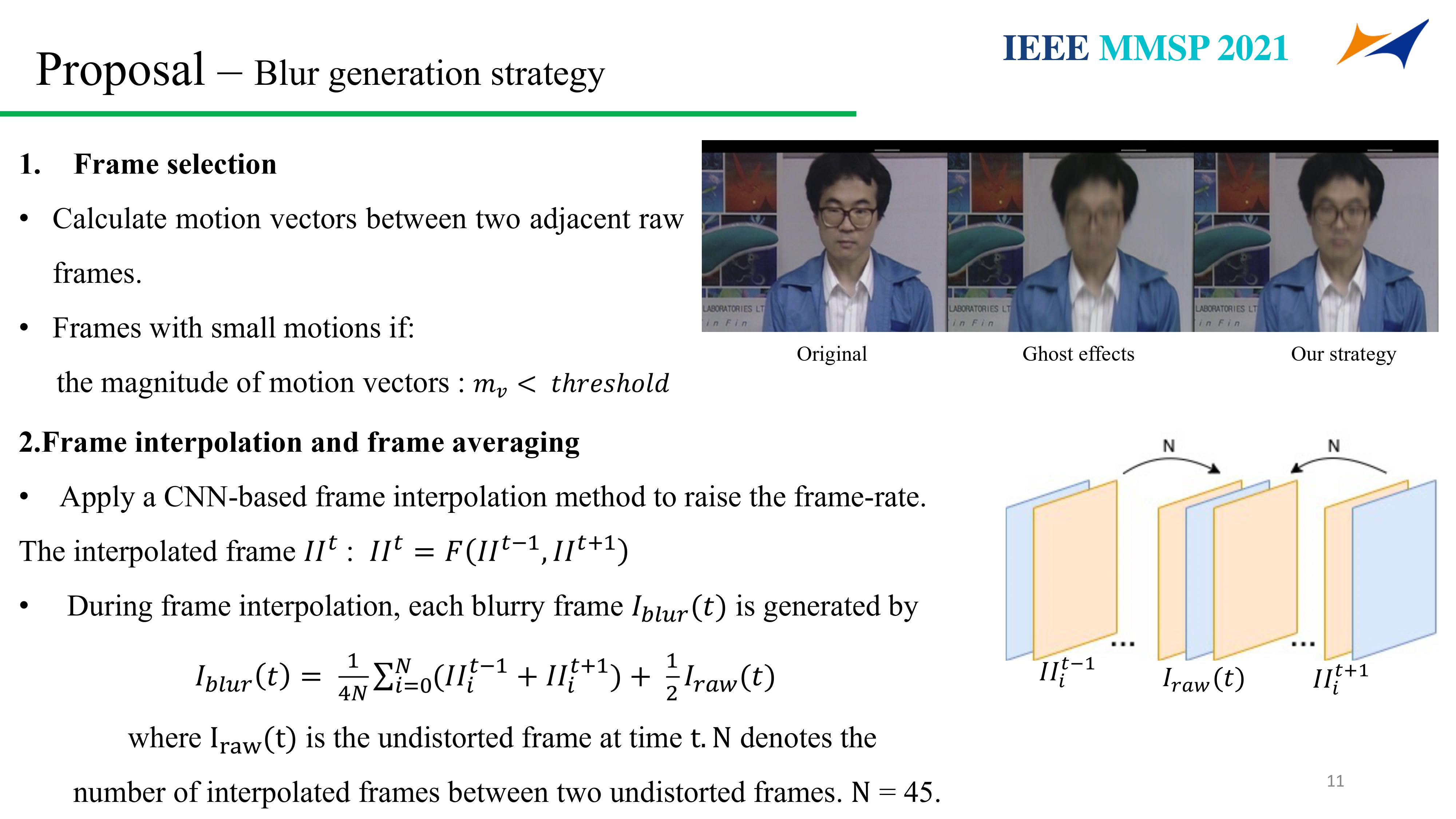
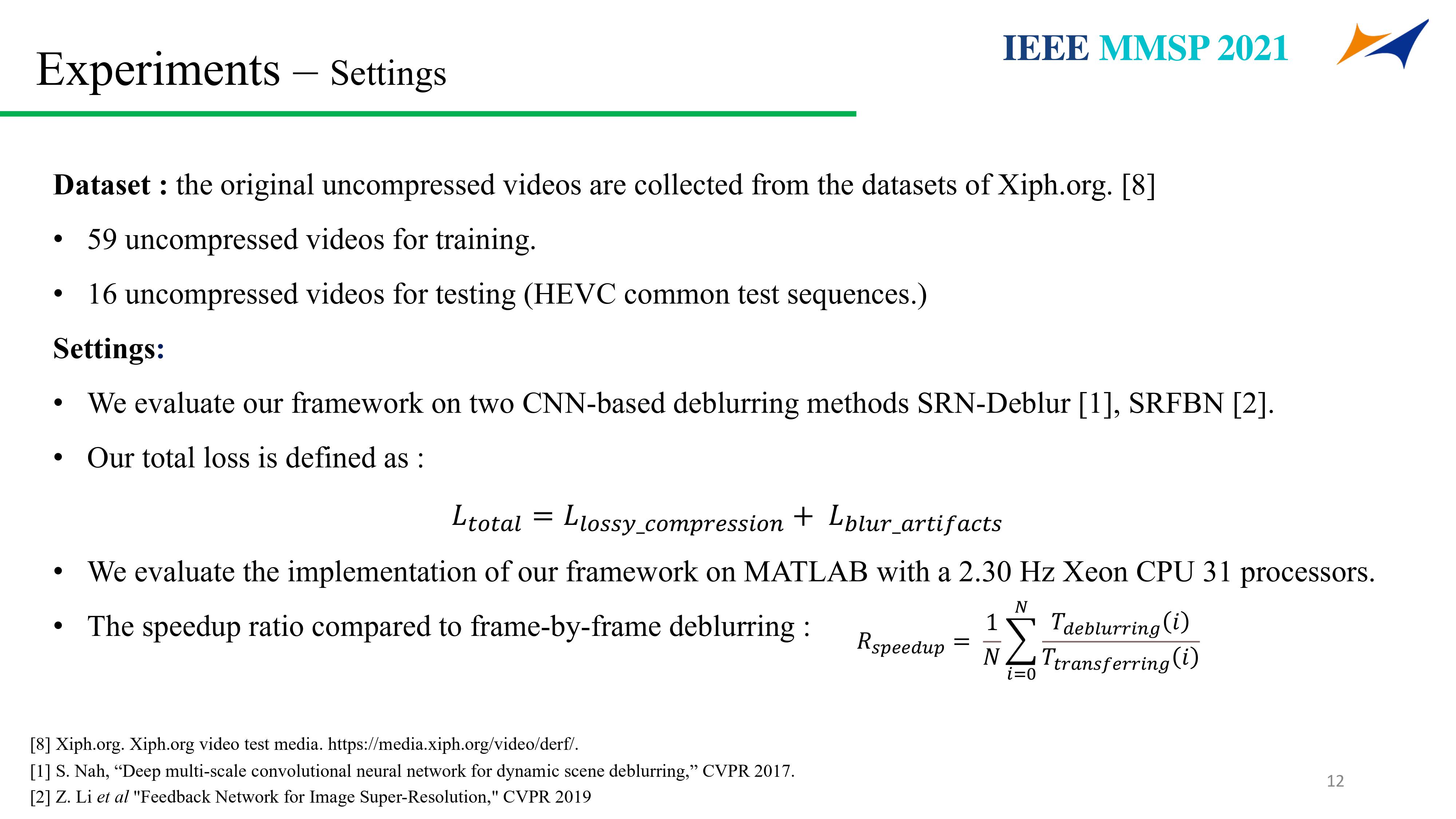
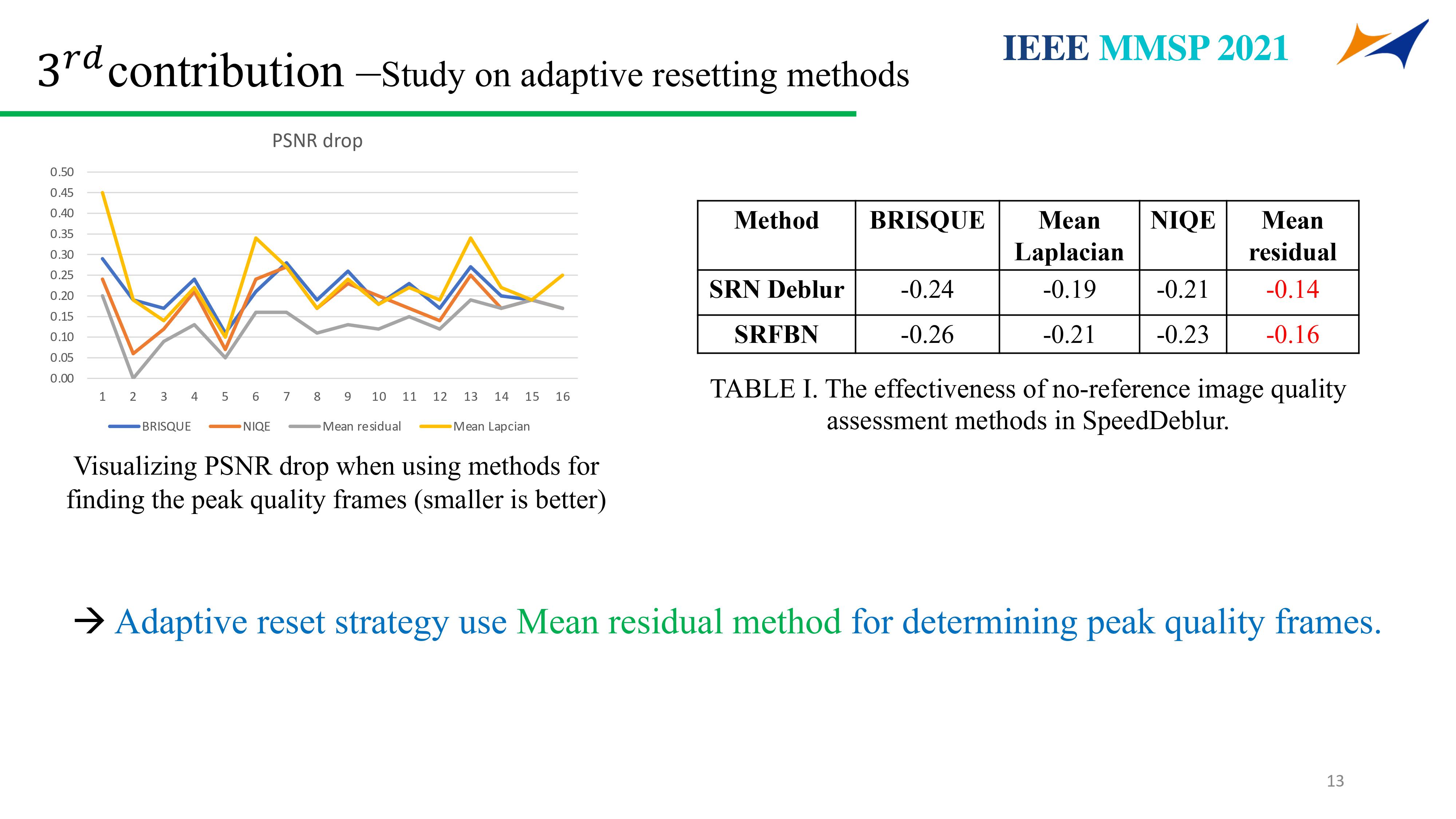
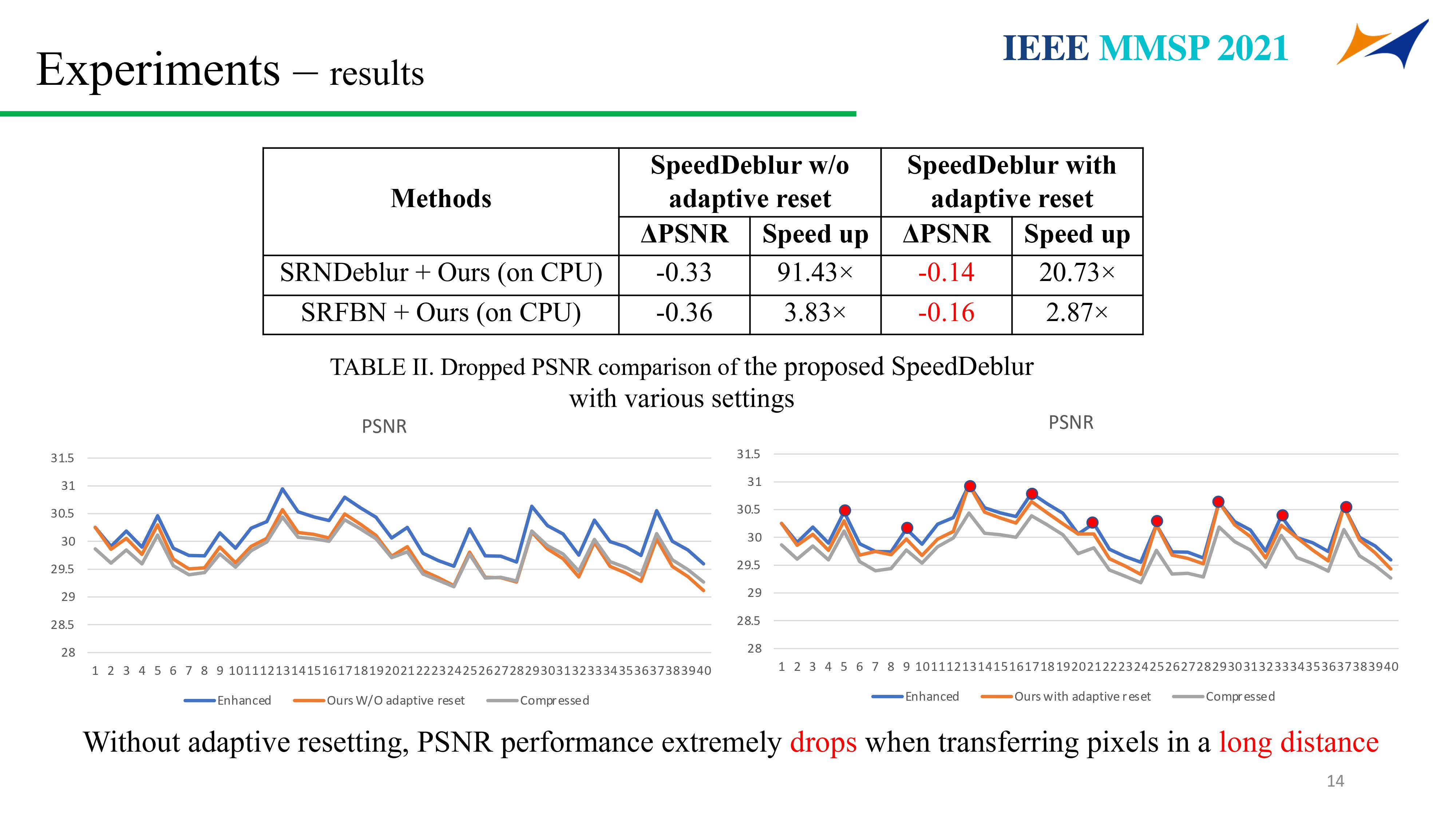
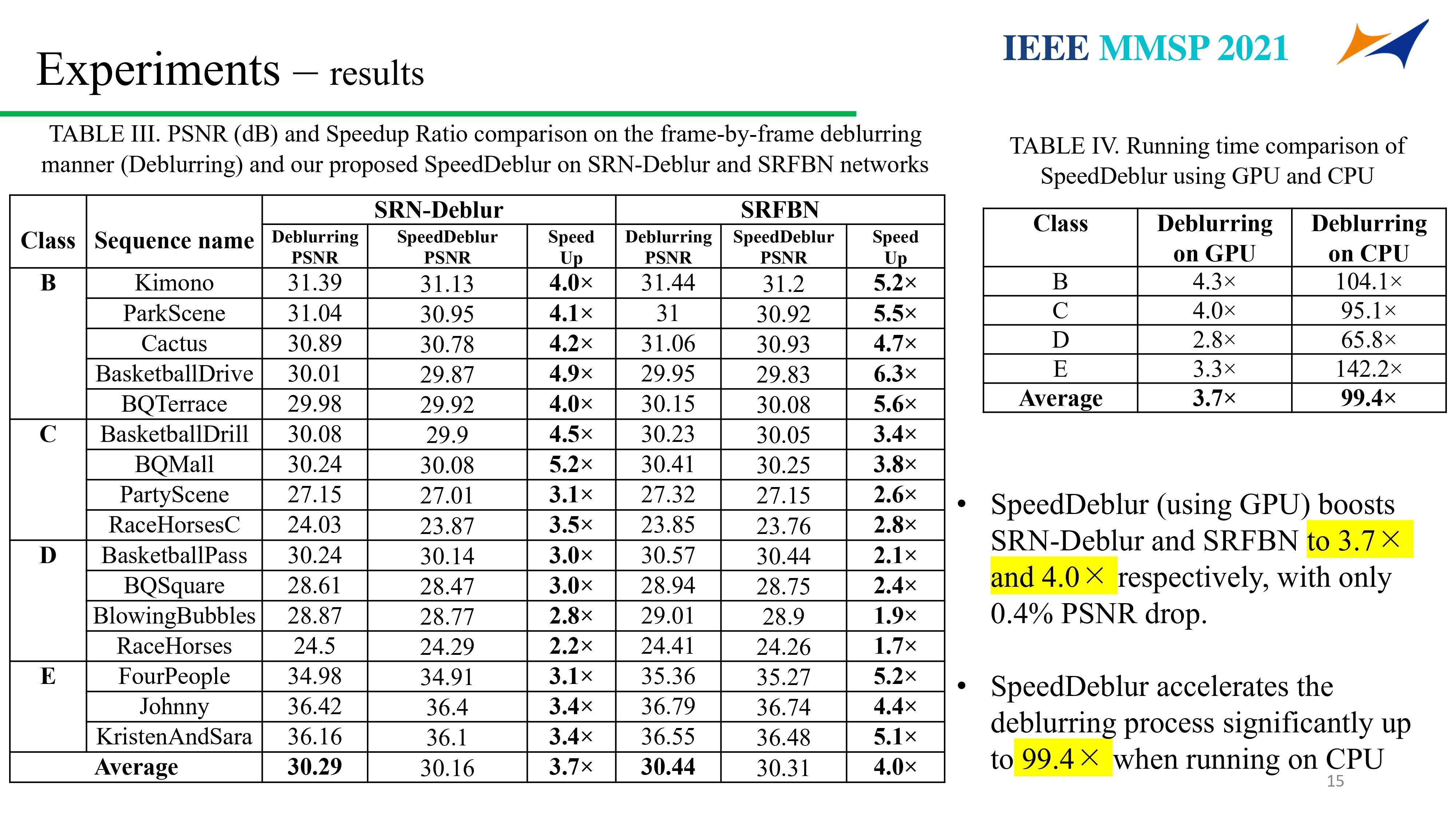
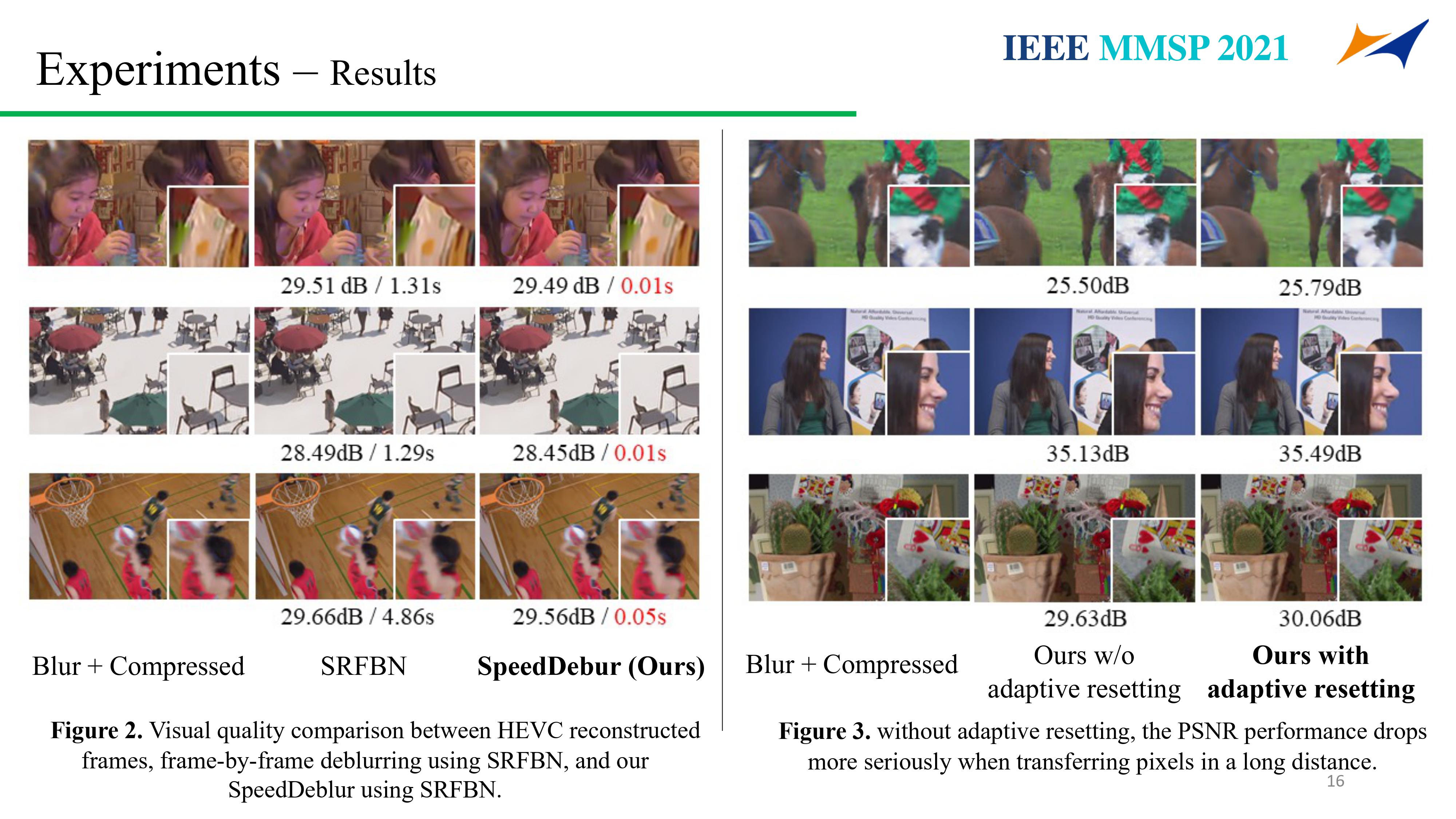
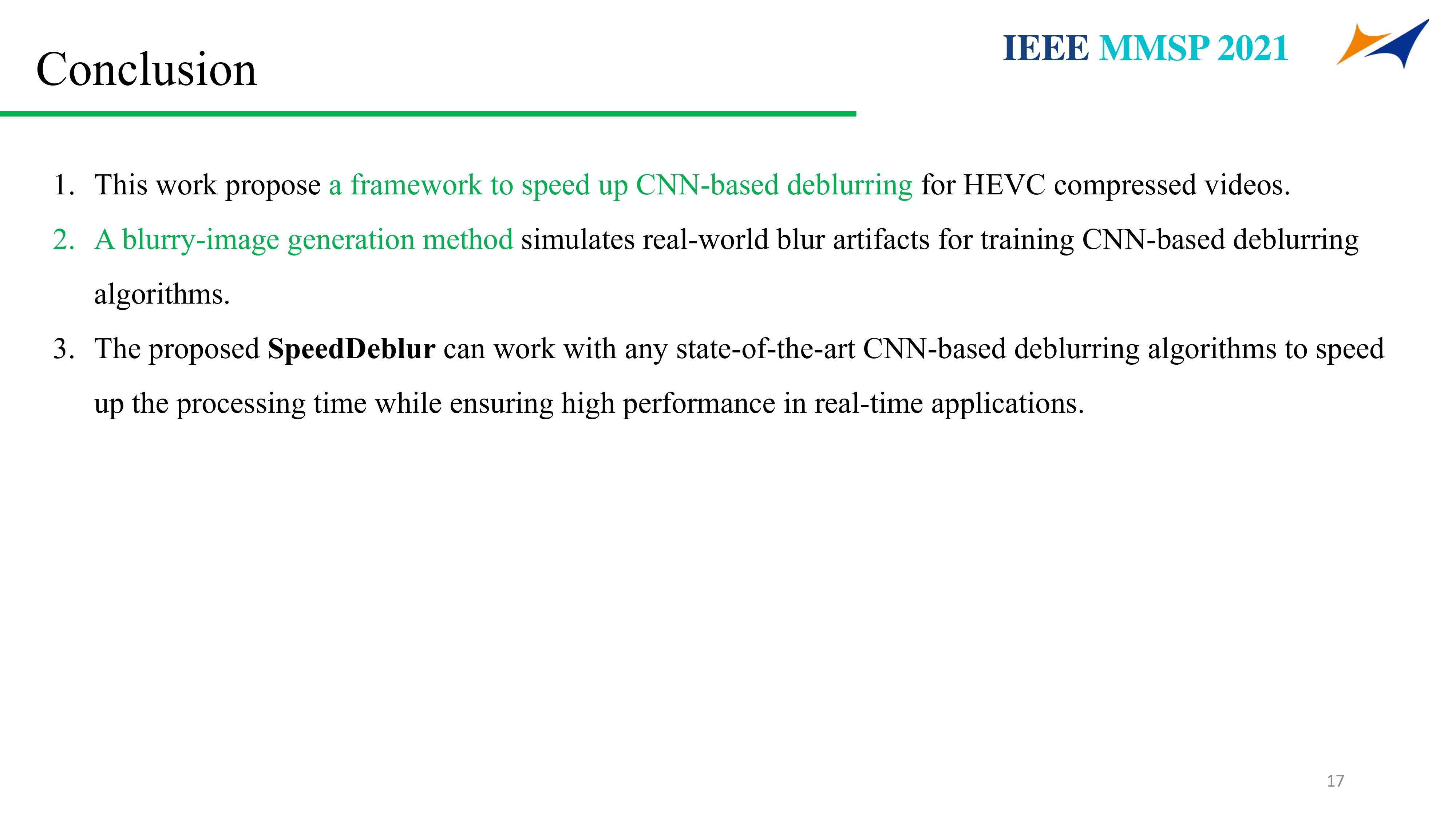
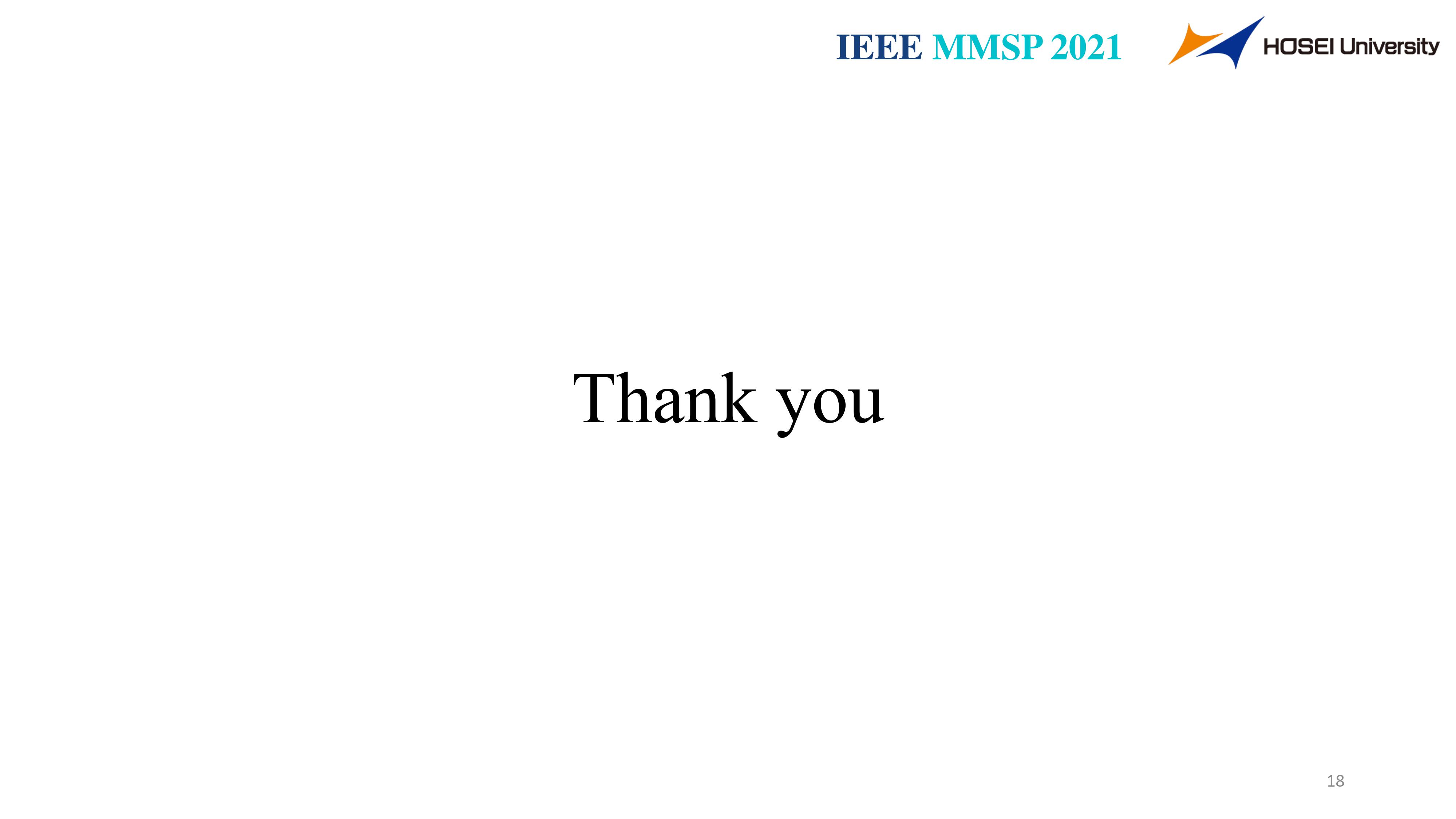
Supervisor
![]() |
Jinjia ZHOU (周 金佳)
Associate Professor, Faculty of Science and Engineering Department of Applied Informatics, Hosei University, Japan. |